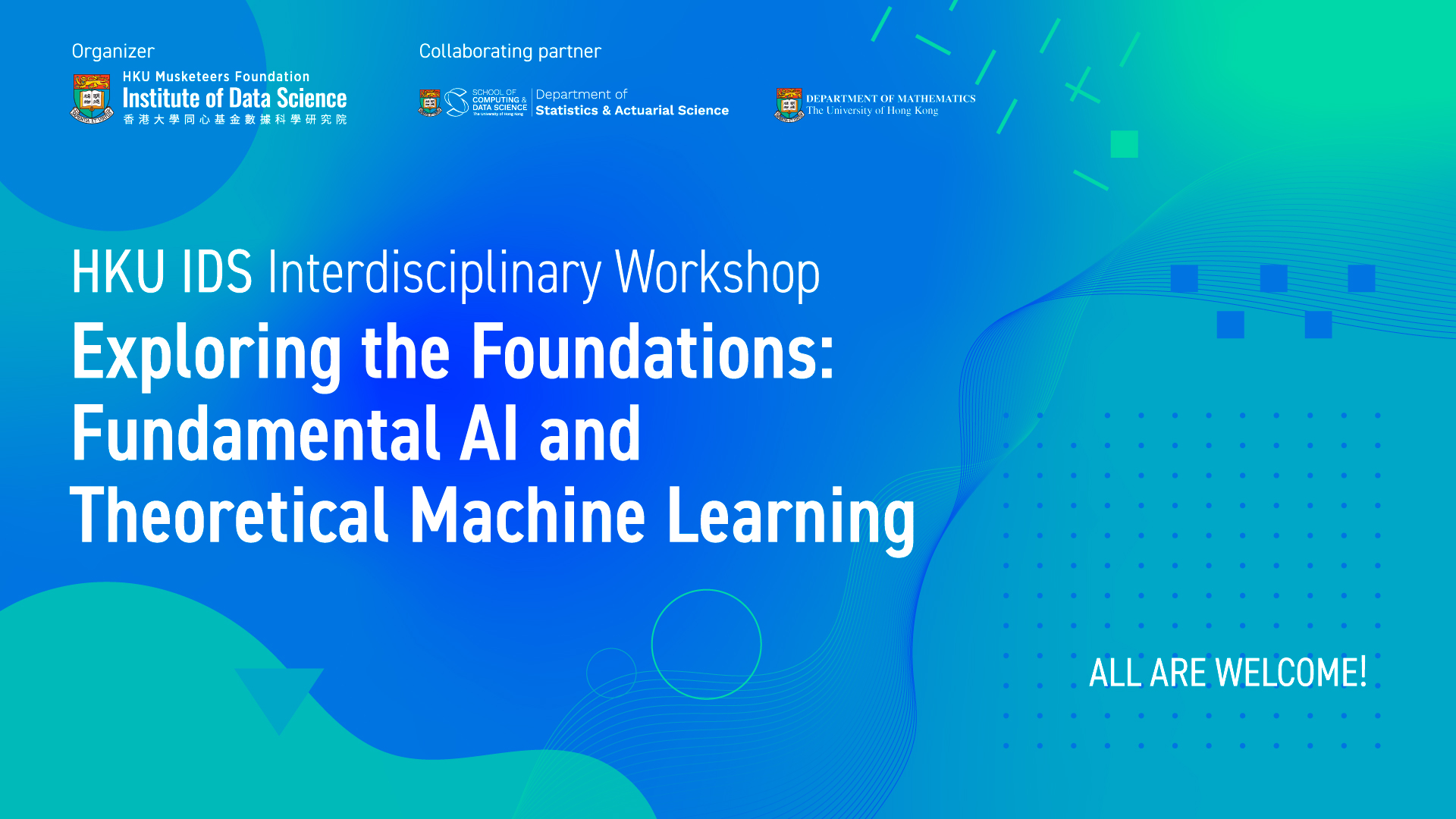
Organizer
Collaborating partner
&
Get ready to dive into the fascinating world of AI and machine learning at the HKU IDS Interdisciplinary Workshop – Exploring the Foundations: Fundamental AI and Theoretical Machine Learning! Mark your calendars for May 28-29, 2025, because this is an event you won’t want to miss.
As AI and machine learning continue to revolutionize our daily lives and academic pursuits, it’s time to spark some serious curiosity and excitement, especially within the research community. This workshop serves as a valuable platform for faculty members and researchers from different units who work on related data science topics to share their novel ideas.
We will expect a vibrant exchange of thoughts and a chance to peek into each other’s projects, all while fostering new connections and collaborations for future research. Whether you’re a seasoned expert or a curious newcomer, this event promises to be a melting pot of innovation and inspiration.
Co-organized with the Department of Statistics and Actuarial Science and the School of Computing and Data Science and the Department of Mathematics, at HKU, this workshop is set to be an exciting journey into the heart of AI and machine learning.
So, pack your intellectual curiosity and join us on campus for the two-day exploration, learning, and fun!
Date
28 – 29 May, 2025 (Wed – Thu)
Venue
1/F – MWT6, Meng Wah Complex, Main Campus, HKU
Invited Speakers
Prof Anru ZHANG
Associate Professor
Department of Biostatistics & Bioinformatics and Department of Computer Science
Duke University
Prof Guodong LI
Associate Director,
(Recruitment and Research),
HKU IDS
Associate Head (Research) & Professor,
SAAS, CDS
The University of Hong Kong
Prof Guodong LI
Associate Director,
(Recruitment and Research),
HKU IDS
Associate Head (Research) & Professor,
SAAS, CDS
The University of Hong Kong
Organizing Committee
Chairman
Prof Guodong LI
Associate Director,
(Recruitment and Research),
HKU Musketeers Foundation Institute of Data Science
Associate Head (Research) & Professor,
Department of Statistics and Actuarial Science
School of Computing and Data Science
School of Computing and Data Science
Prof Long FENG
Assistant Professor
Department of Statistics and Actuarial Science
School of Computing and Data Science
School of Computing and Data Science
Prof Yingyu LIANG
Associate Professor
HKU Musketeers Foundation Institute of Data Science
Department of Computer Science, School of Computing and Data Science
Prof Yuan CAO
Assistant Professor
Department of Statistics and Actuarial Science
School of Computing and Data Science
School of Computing and Data Science
Dr Wenjie HUANG
Research Assistant Professor
HKU Musketeers Foundation Institute of Data Science
Department of Data and Systems Engineering
HKU Speakers
Prof Yi MA
Director of HKU Musketeers Foundation Institute of Data Science
Director of HKU School of Computing and Data Science
Professor, Chair of Artificial Intelligence
Prof Yuan CAO
Assistant Professor
Department of Statistics and Actuarial Science
School of Computing and Data Science
School of Computing and Data Science
Dr Wenjie HUANG
Research Assistant Professor
HKU Musketeers Foundation Institute of Data Science
Department of Data and Systems Engineering
Dr Yue XIE
Research Assistant Professor
HKU Musketeers Foundation Institute of Data Science
Department of Mathematics
Prof Difan ZOU
Assistant Professor
HKU Musketeers Foundation Institute of Data Science
School of Computing and Data Science
HKU Musketeers Foundation Institute of Data Science
School of Computing and Data Science
Programme (Tentative)
28 May, 2025 (Wed)
Morning Session
08:30 – 09:00 |
Registration |
09:00 – 09:15 |
Opening Remarks by Prof Yi MAThe University of Hong Kong |
09:15 – 10:05 |
Prof Anru ZHANGDuke UniversitySmooth Flow MatchingAbstractFunctional data, i.e., smooth random functions observed over continuous domains, are increasingly common in fields such as neuroscience, health informatics, and epidemiology. However, privacy constraints, sparse/irregular sampling, and non-Gaussian structures present significant challenges for generative modeling in this context. In this work, we propose Smooth Flow Matching, a new generative framework for functional data that overcomes these challenges. Built upon flow matching ideas, SFM constructs a smooth three-dimensional vector field to generate infinite-dimensional functional data, without relying on Gaussianity or low-rank assumptions. It is computationally efficient, handles sparse and irregular observations, and guarantees smoothness of the generated functions, offering a practical and flexible solution for generative modeling of functional data. |
10:05 – 10:55 |
Prof Yingyu LIANGThe University of Hong Kong
Can Language Models Compose Skills Demonstrated In-Context?AbstractThe ability to compose basic skills to accomplish composite tasks is believed to be a key for reasoning and planning in intelligent systems. In this work, we propose to investigate the \emph{in-context composition} ability of language models: the model is asked to perform a composite task that requires the composition of some basic skills demonstrated only in the in-context examples. This is more challenging than the typical setting where the basic skills and their composition can be learned in the training time. We perform systematic empirical studies using example language models on linguistic and logical composite tasks. The experimental results show that they in general have limited in-context composition ability due to the failure to recognize the composition and identify proper skills from in-context examples, even with the help of Chain-of-Thought examples. We also provide a theoretical analysis in stylized settings to show that proper retrieval of the basic skills for composition can help the composite tasks. Based on the insights, we propose a new method, Expanded Chain-of-Thought, which converts basic skill examples into composite task examples with missing steps to facilitate better utilization by the model. The method leads to significant performance improvement, which verifies our analysis and provides inspiration for future algorithm development. |
10:55 – 11:10 |
Tea & Coffee Break |
11:10 – 12:00 |
Prof Atsushi SUZUKIThe University of Hong Kong Hallucinations are inevitable but statistically negligibleAbstractHallucinations, a phenomenon where a language model (LM) generates nonfactual content, pose a significant challenge to the practical deployment of LMs. While many empirical methods have been proposed to mitigate hallucinations, a recent study established a computability-theoretic result showing that any LM will inevitably generate hallucinations on an infinite set of inputs, regardless of the quality and quantity of training datasets and the choice of the language model architecture and training and inference algorithms. Although the computability-theoretic result may seem pessimistic, its significance in practical viewpoints has remained unclear. In contrast, we present a positive theoretical result from a probabilistic perspective. Specifically, we prove that hallucinations can be made statistically negligible, provided that the quality and quantity of the training data are sufficient. Interestingly, our positive result coexists with the computability-theoretic result, implying that while hallucinations on an infinite set of inputs cannot be entirely eliminated, their probability can always be reduced by improving algorithms and training data. By evaluating the two seemingly contradictory results through the lens of information theory, we argue that our probability-theoretic positive result better reflects practical considerations than the computability-theoretic negative result. |
Afternoon Session
14:00 – 14:50 |
Prof Yiqiao ZHONGUniversity of Wisconsin–MadisonCan large language models solve compositional tasks? A study of out-of-distribution generalizationAbstractLarge language models (LLMs) such as GPT-4 sometimes appeared to be creative, solving novel tasks with a few demonstrations in the prompt. These tasks require the pre-trained models to generalize on distributions different from those from training data—which is known as out-of-distribution generalization. For example, in “symbolized language reasoning” where names/labels are replaced by arbitrary symbols, yet the model can infer the names/labels without any finetuning. In this talk, I will focus on a pervasive structure within LLMs known as induction heads. By experimenting on a variety of LLMs, I will empirically demonstrate that compositional structure is crucial for Transformers to learn the rules behind training instances and generalize on OOD data. Further, I propose the “common bridge representation hypothesis” where a key intermediate subspace in the embedding space connects components of early layers and those of later layers as a mechanism of composition. |
14:50 – 15:40 |
Prof Long FENGThe University of Hong Kong A Nonparametric Statistics Approach to Feature Selection in Deep Neural NetworksAbstractFeature selection is a classic statistical problem that seeks to identify a subset of features that are most relevant to the outcome. In this talk, we consider the problem of feature selection in deep neural networks. Unlike typical optimization-based deep learning methods, we formulate neural networks into index models and propose to learn the target set using the second-order Stein’s formula. Our approach is not only computationally efficient by avoiding the gradient-descent-type algorithm for solving highly nonconvex deep-learning-related optimizations, but more importantly, it can theoretically guarantee variable selection consistency for deep neural networks when the sample size $n = \Omega(p^2)$, where $p$ is the dimension of the input. Comprehensive simulations and real genetic data analyses further demonstrate the superior performance of our approach. |
15:40 – 15:55 |
Tea & Coffee Break |
15:55 – 16:45 |
Prof Difan ZOUThe University of Hong Kong
|
16:45 – 17:35 |
Dr Yue XIEThe University of Hong Kong |
29 May, 2025 (Thu)
Morning Session
09:00 – 09:50 |
Prof Guodong LI
The University of Hong Kong
Unraveling Recurrent Dynamics: How Neural Networks Model Sequential DataAbstractThe long-proven success of recurrent models in handling sequential data has triggered researchers to explore its statistical explanations. Yet, a fundamental question remains unaddressed: What elementary temporal patterns can these models capture at a granular level? This paper answers this question by discovering the underlying basic features of recurrent networks’ dynamics through an intricate mathematical analysis. Specifically, by block-diagonalizing recurrent matrices via real Jordan decomposition, we successfully decouple the recurrent dynamics into a collection of elementary patterns, yielding a new concept of recurrence features. It is further demonstrated by empirical studies that the recurrent dynamics in sequential data are mainly dominated by low-order recurrence features. This motivates us to consider a parallelized network comprising small-sized units, each having as few as two hidden states. Compared to the original network with a single large-sized unit, it accelerates computation dramatically while achieving comparable performance. |
09:50 – 10:40 |
Prof Yuan CAOThe University of Hong Kong
|
10:40 – 10:55 |
Tea & Coffee Break |
10:55 – 11:45 |
Prof Yunwen LEIThe University of Hong Kong Stochastic Gradient Methods: Bias, Stability and GeneralizationAbstractRecent developments of stochastic optimization often suggest biased gradient estimators to improve either the robustness, communication efficiency or computational speed. Representative biased stochastic gradient methods (BSGMs) include Zeroth-order stochastic gradient descent (SGD), Clipped-SGD and SGD with delayed gradients. In this talk, we present the first framework to study the stability and generalization of BSGMs for convex and smooth problems. We apply our general result to develop the first stability bound for Zeroth-order SGD with reasonable step size sequences, and the first stability bound for Clipped-SGD. While our stability analysis is developed for general BSGMs, the resulting stability bounds for both Zeroth-order SGD and Clipped-SGD match those of SGD under appropriate smoothing/clipping parameters. |
11:45 – 12:35 |
Dr Wenjie HUANGThe University of Hong Kong The role of mixed discounting in risk-averse sequential decision-makingAbstractThis work proposes a new principled constructive model for risk preference mapping in infinite-horizon cash flow analysis. The model prescribes actions that account for both a traditional discounting by scaling the future incomes and a random interruption time for the cash flow. Data from an existing field experiment provides evidence that supports the use of our proposed mixed discounting model in place of the more traditional one for a significant proportion of participants, i.e. 30% of them. This proportion climbs above 80% when enforcing the use of more reasonable discount factors. On the theoretical side, we shed light on some properties of the new preference model, establishing conditions under which the infinite-horizon risk is finite, and conditions where the mixed discounting model can be seen as either equivalent or providing a bound on the risk perceived by the traditional approach. Finally, an illustrative example on optimal stopping problem shows impacts of employing our mixed discounting model on the optimal threshold policy. |
12:35 – 12:45 |
Closing Remarks |
For enquiry, please contact us at datascience@hku.hk.