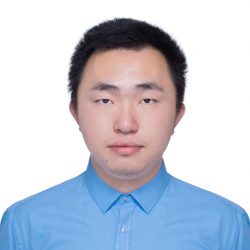
Dr. Fei Jing is a Post-doctoral Research Fellow at the HKU Musketeers Foundation Institute of Data Science (HKU IDS), working alongside Dr. Qingpeng Zhang. Fei obtained his PhD and BS in Mathematics and Applied Mathematics, both from the Shandong University. His research interests broadly lie in the interdisciplinary applications of mathematics, complex networks and machine learning. In particular, he works on applying mathematical tools such as probability and statistical methods, differential equations, and discrete analysis to machine learning interpretability and data analysis.
Currently, his present focus is on delineating the intricate relationship between data structures and the boundary performance in binary classification tasks.
Traditional machine learning models have historically relied on error-centric metrics for objective functions. However, it has become increasingly clear that such metrics may not significantly influence optimization results and can be particularly problematic in the face of imbalanced datasets, a common hurdle in classification tasks. This realization has catalyzed a shift towards prioritizing Area Under the Curve (AUC) optimization in classification problems, giving rise to a diverse array of new learning paradigms, methodological advancements, and optimization strategies. These innovations have been effectively applied across various domains. Alongside practical developments, there have been theoretical strides made in understanding model consistency and limiting generalization errors. At the heart of this evolution is binary classification, a core machine learning endeavor that categorizes subjects into two separate groups. It’s a pervasive challenge with wide-ranging relevance. This project aims to illuminate the elusive relationship between the intrinsic patterns of data and the consequent performance of binary classifiers. It’s an exploration to establish a measurable connection that has, until now, been veiled in complexity.
- Jing F, Liu C, Wu J L, et al. Toward structural controllability and predictability in directed networks[J]. IEEE Transactions on Systems, Man, and Cybernetics: Systems, 2022, 52(12): 7692-7701.
Network science; Complex systems; Combinatorial mathematics; Machine learning; Drug discovery.