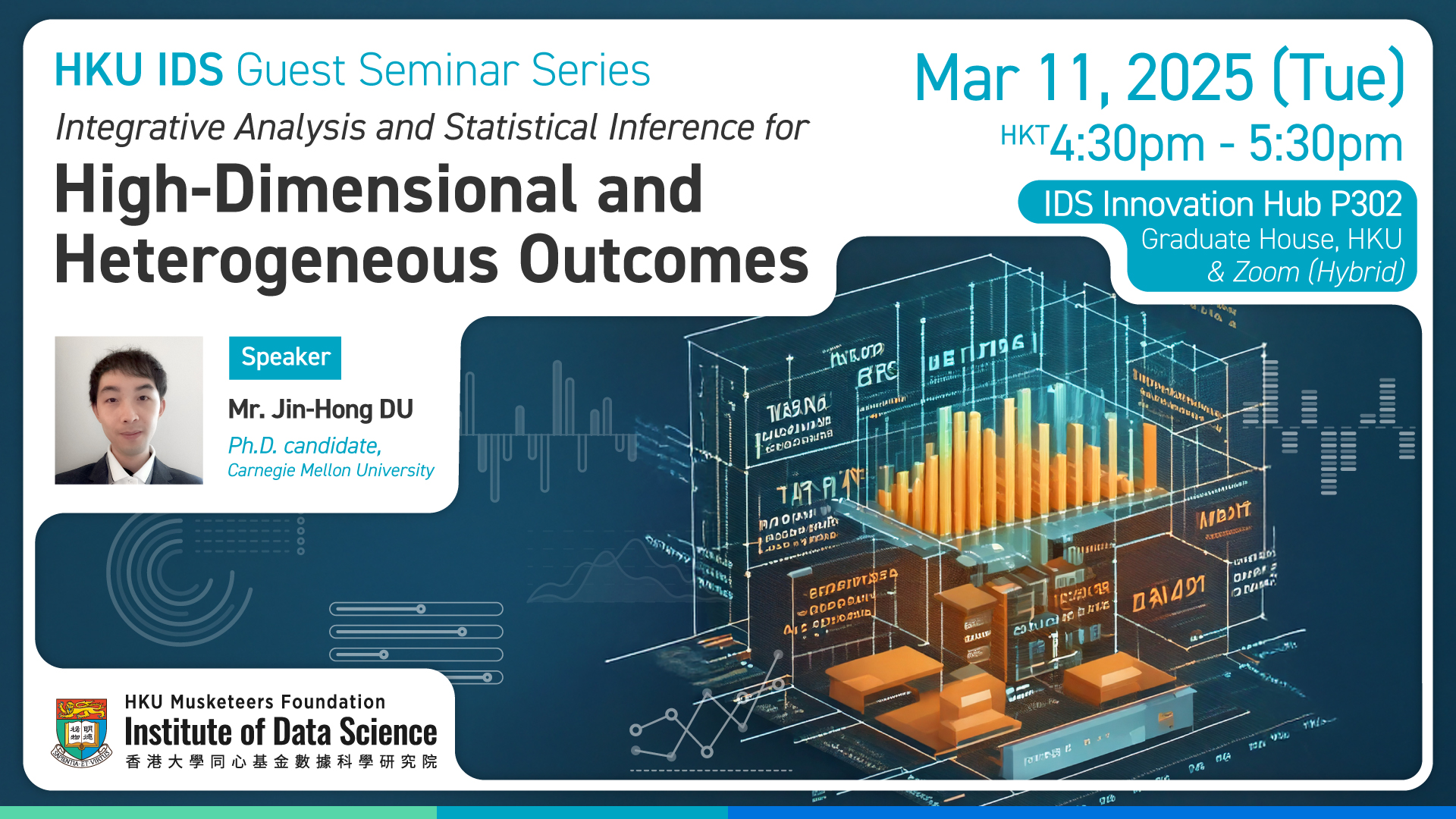
IDS Guest Seminar - by Mr. Jin-Hong Du
Title: Integrative Analysis and Statistical Inference for High-Dimensional and Heterogeneous Outcomes
Speaker: Mr. Jin-Hong Du, Ph.D. candidate, Carnegie Mellon University
Date: Mar 11, 2025
Time: 4:30pm – 5:30pm
Venue: Room P302, HKU IDS Innovation Hub, Graduate House, HKU / Zoom
Mode: Hybrid. Seats for on-site participants are limited. A confirmation email will be sent to participants who have successfully registered.
Abstract
Single-cell genomics has revolutionized our understanding of cellular heterogeneity, but it also presents unique statistical challenges. The high-dimensional, sparse, and overdispersed nature of single-cell count data, coupled with complex batch effects and unmeasured covariates, necessitates sophisticated analytical approaches. Data integration methods have been developed to address these challenges by extracting low-dimensional embeddings from high-dimensional outcomes to remove unwanted variations across heterogeneous datasets. However, performing multiple hypothesis testing after integration can introduce bias due to data-dependent processes.
In this talk, I will present statistical tools I have developed for robust inference with high-dimensional heterogeneous outcomes in single-cell data analysis, specifically addressing the challenge of hidden variations. These methods are applicable to two key areas: subject-level pseudo-bulk differential expression analysis and single-cell CRISPR perturbation effect analysis. I will discuss two distinct deconfounding strategies that are flexible to account for hidden confounders, mediators, and moderators. The first method leverages sparse effects to bypass the estimation of hidden variations, while the second utilizes negative control outcomes to directly recover unmeasured variations. These approaches not only address the biases in multiple hypothesis testing after data integration but also enhance the reliability and power of statistical inferences in single-cell studies.
Speaker
Jin-Hong is a fourth-year PhD candidate in Statistics and Machine Learning at Carnegie Mellon University, where he is advised by Professors Kathryn Roeder and Larry Wasserman. His research focuses on tackling the statistical and machine learning challenges associated with single-cell multiomics and CRISPR perturbation analysis. He develops data-adaptive statistical methods that ensure valid statistical and causal inference while leveraging machine learning techniques to facilitate reliable discoveries in high-dimensional heterogeneous data.
For information, please contact:
Email: datascience@hku.hk