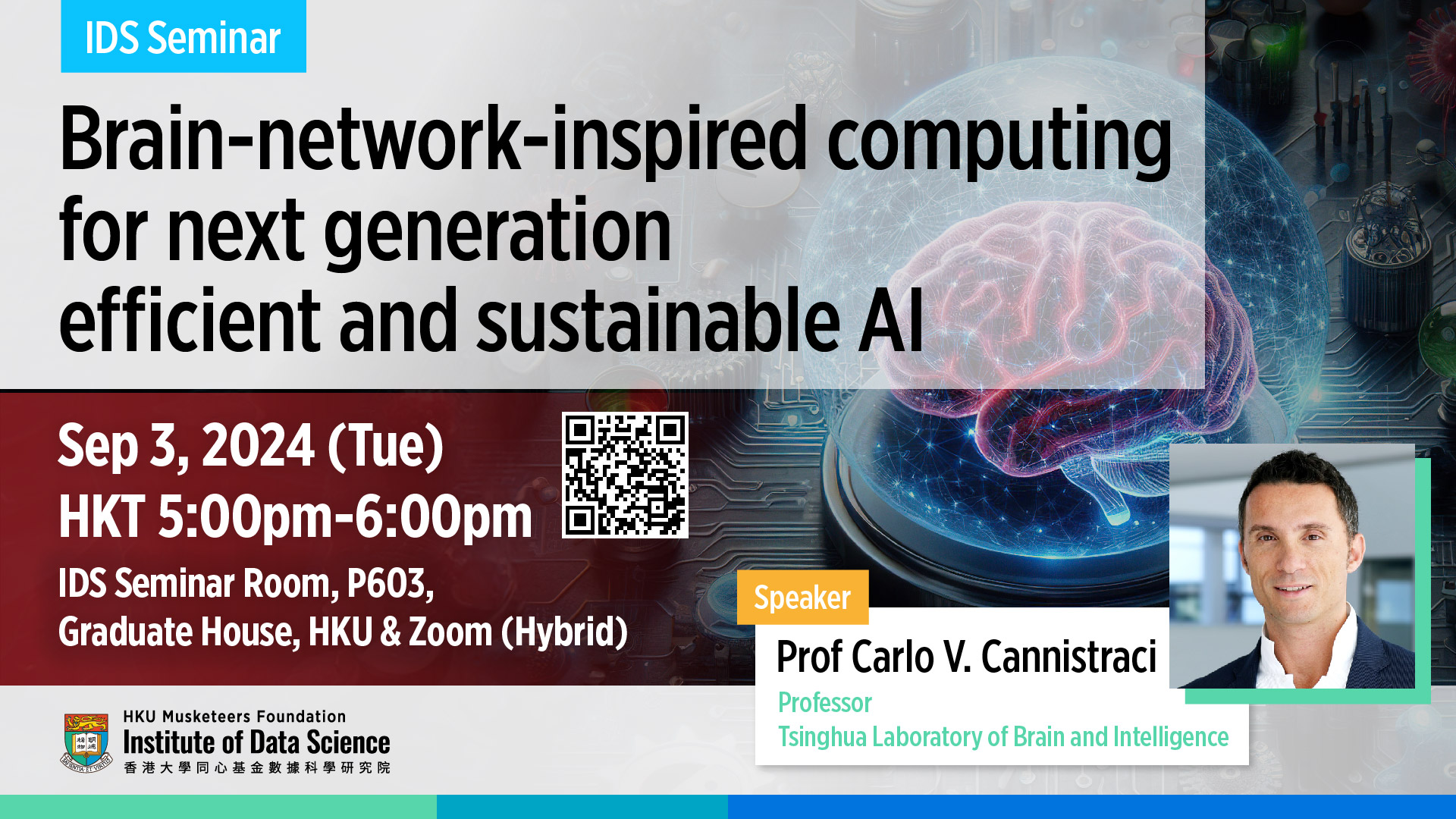
IDS Seminar: Brain-network-inspired computing for next generation efficient and sustainable AI
Venue: IDS Seminar Room, P603, Graduate House / Zoom
Mode: Hybrid. Seats for on-site participants are limited. A confirmation email will be sent to participants who have successfully registered.
Abstract
The human brain is remarkably efficient, learning with just a few watts. In contrast, today’s large language models are voracious power consumers, demanding over 100 times the power expenditure of the human brain. Brain-inspired network science research can play a relevant role for designing low-consumption and efficient deep learning. We need to develop new concepts and theories for an ecological and sustainable approach to AI, and some of these new computing paradigms can be borrow from the brain and its complex systems biology.
In the Center for Complex Network Intelligence (CCNI), which I lead at the Tsinghua Laboratory of Brain and Intelligence (THBI), we are interested to investigate three key brain network features adopted for efficient computing: connectivity sparsity, connectivity morphology and neuro-glia coupling.
In this talk, I will present the results that we have achieved this year. On sparsity, our study “Epitopological learning and Cannistraci-Hebb network shape intelligence brain-inspired theory for ultra-sparse advantage in deep learning”1 is published in The Twelfth International Conference on Learning Representations (ICLR) 2024. On sparse morphology, our study “Neuromorphic dendritic network computation with silent synapses for visual motion perception”2 is published in Nature Electronics.
References
- Epitopological Learning and Cannistraci-Hebb Network Shape Intelligence Brain-Inspired Theory for Ultra-Sparse Advantage in Deep Learning. Y Zhang, J Zhao, W Wu, A Muscoloni, CV Cannistraci. The Twelfth International Conference on Learning Representations (ICLR) 2024
- Neuromorphic dendritic network computation with silent synapses for visual motion perception. E Baek, S Song, CK Baek, Z Rong, L Shi, CV Cannistraci. Nature Electronics, 1-12
Speaker
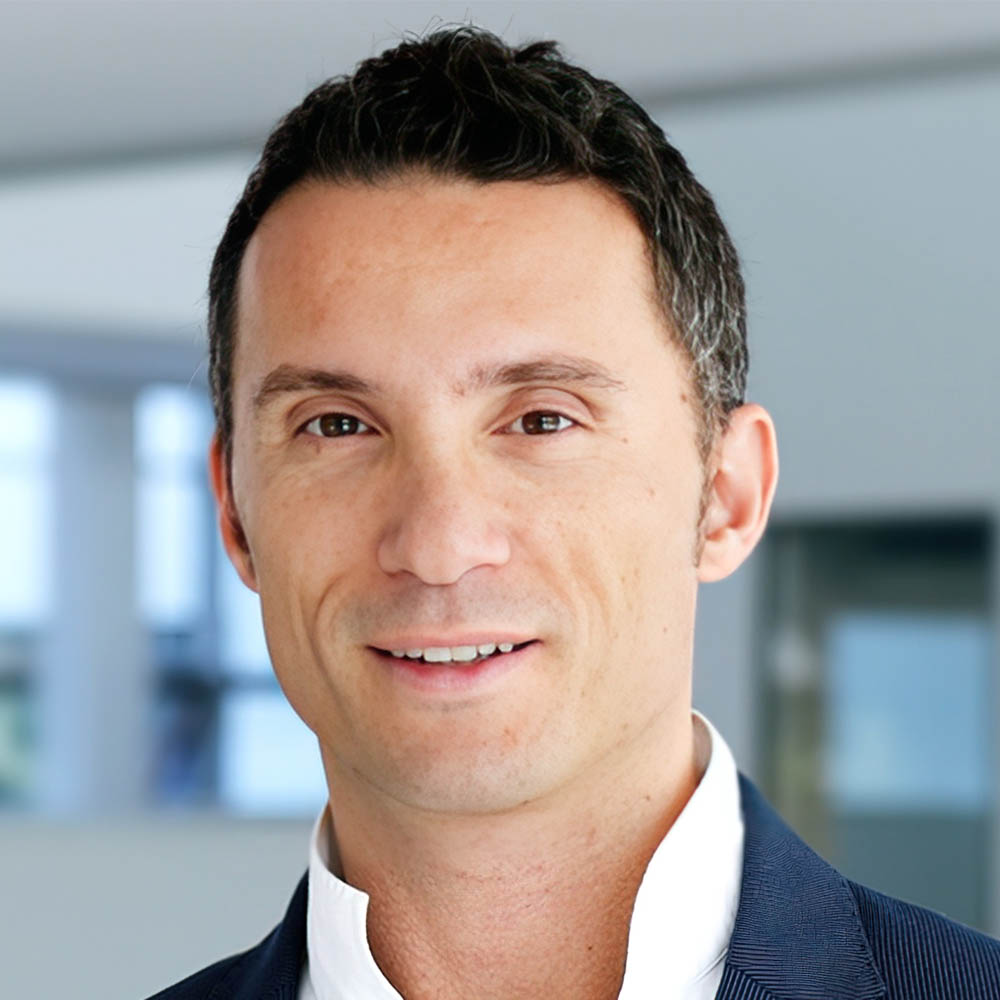
Adjunct Professor @ Department of Computer Science & School of Biomedical Engineering, Tsinghua University
For information, please contact:
Email: datascience@hku.hk
- August 16, 2024
- Events, Gallery, Past Events
- IDS Seminar / Guest Lecture