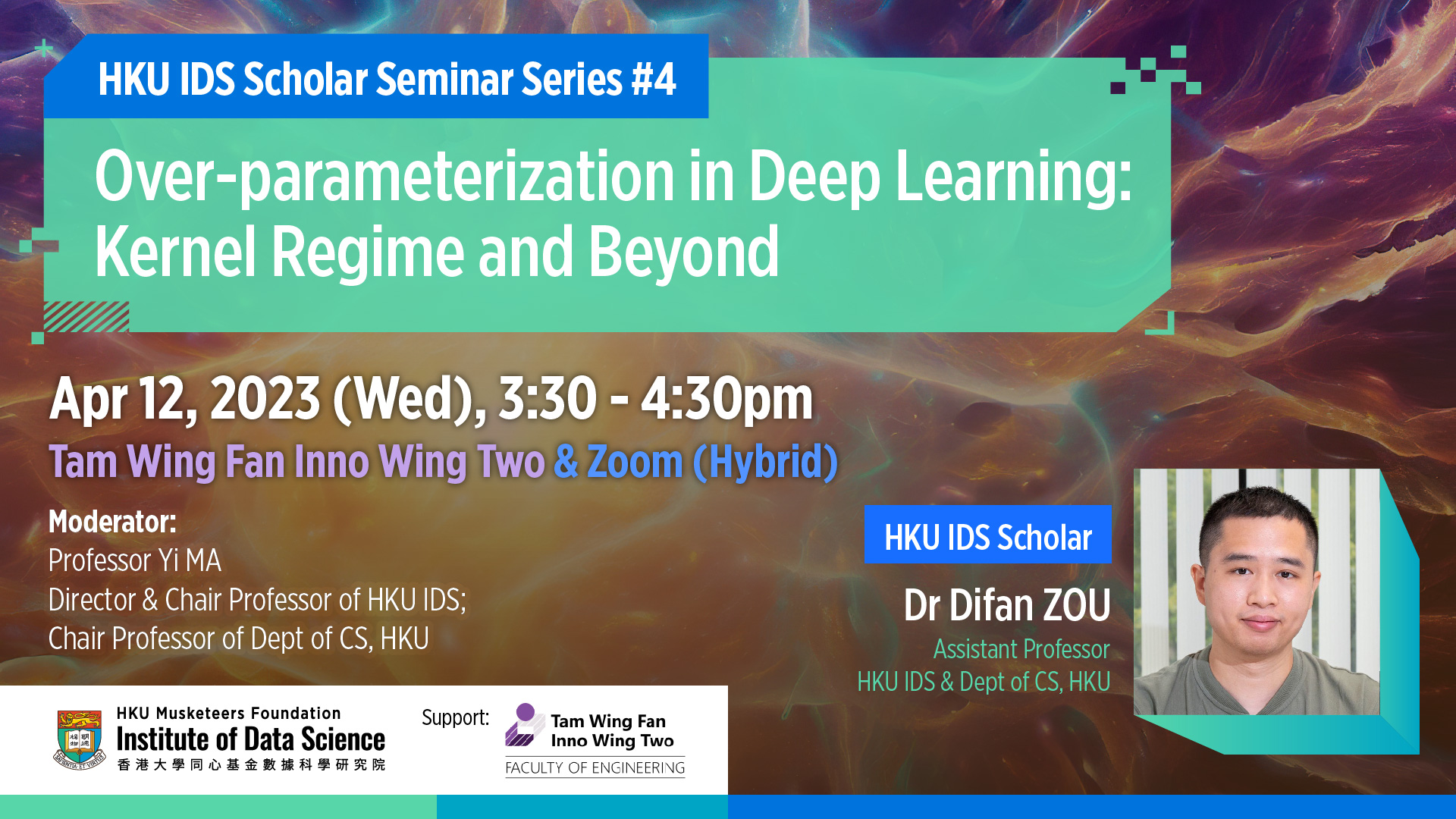
- March 22, 2023
- Events, Gallery
- HKU IDS Scholar Seminar Series
Mode: Hybrid. Seats for on-site participants are limited. A confirmation email will be sent to participants who have successfully registered.
Seminar recording:
Abstract
In recent years, deep learning has revolutionized the field of artificial intelligence, achieving remarkable success in a variety of applications. However, the high-dimensional and nonconvex nature of deep neural networks has made it challenging to understand their behavior and performance. Over-parameterization, which refers to the phenomenon where neural networks have more parameters than necessary to fit the training data, has become a key concept in the study of deep learning. In this talk, the speaker will explore the concept of over-parameterization and introduce a series of recent works that fall in the so-called “kernel regime where the neural network behaves like a kernel method. Furthermore,I will discuss the advantages and limitations of these kernel-based analyses, and introduce several remarkable attempts beyond the kernel regime. Specifically, the speaker will discuss how over-parameterization can affect generalization, optimization, and sample complexity. Overall, this talk aims to provide a comprehensive overview of over-parameterization in deep learning, and to highlight key questions as well as further research directions in this exciting and rapidly evolving area.
Speaker
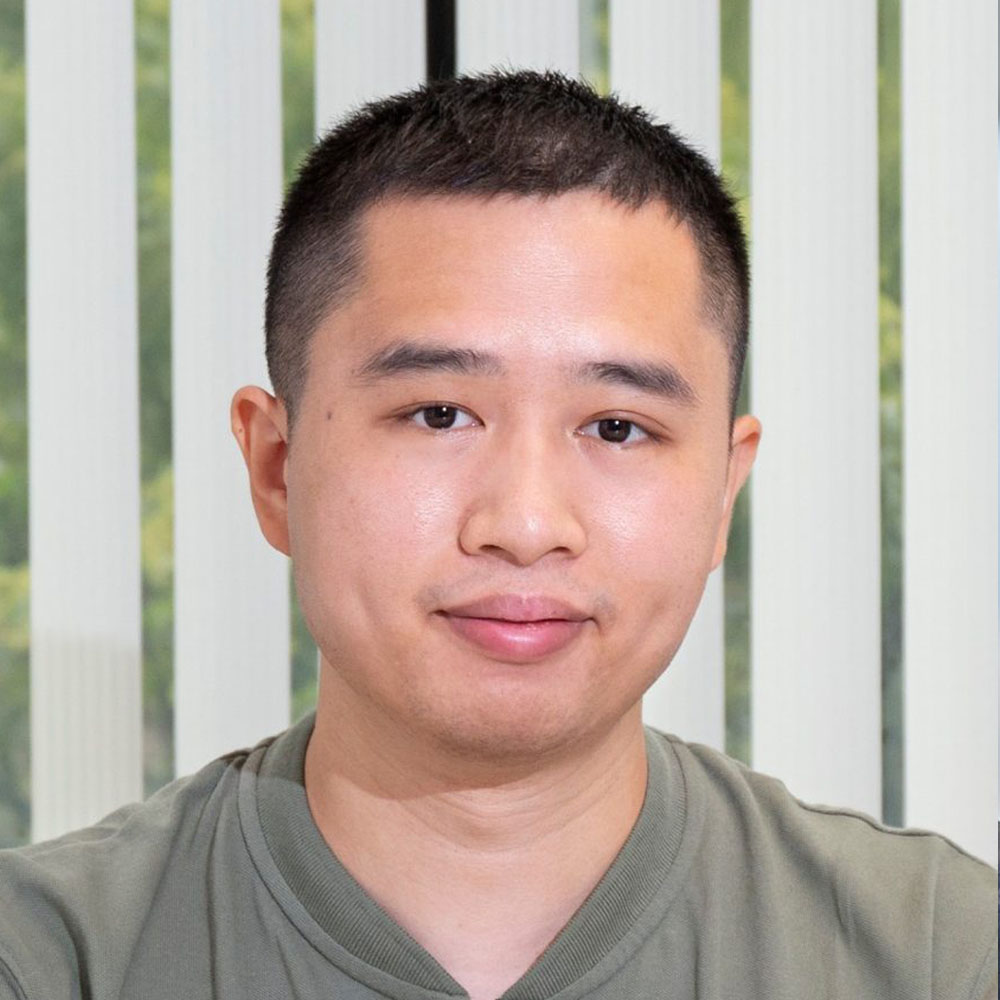
For information, please contact:
Email: datascience@hku.hk