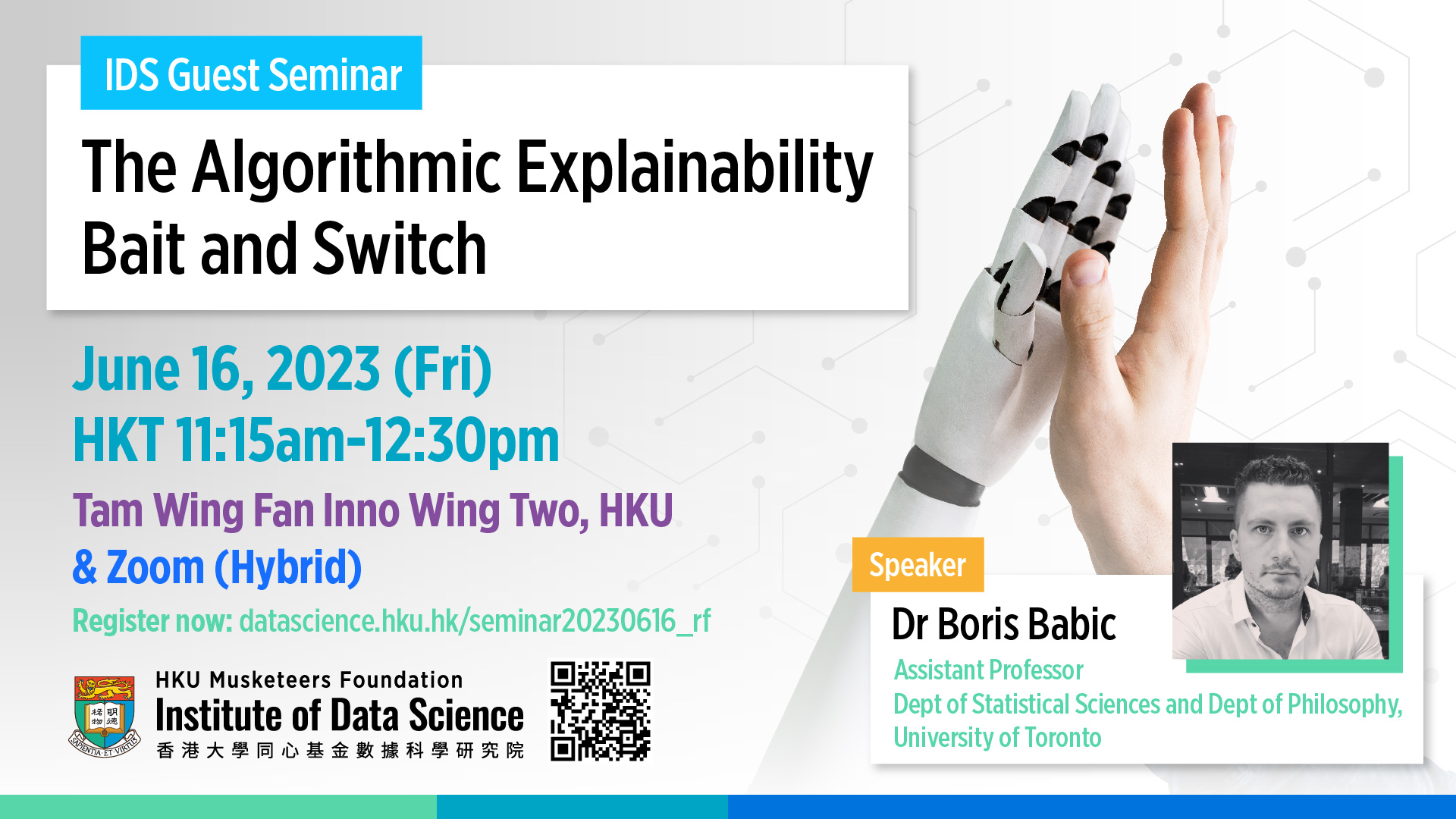
- June 13, 2023
- Events
- IDS Seminar / Guest Lecture
Mode: Hybrid. Seats for on-site participants are limited. A confirmation email will be sent to participants who have successfully registered.
Abstract
Explainability in machine learning (ML) is emerging as a leading area of academic research and a topic of significant legal and regulatory concern. Indeed, a near-consensus is emerging in favour of explainable ML among lawmakers, academics, and civil society groups. In this project, we challenge this prevailing trend. We argue that explaining ML predictions is at best unnecessary or misleading and at worst socially harmful. Unlike interpretable ML, which we endorse where it is feasible, explainable ML can deliver on none of the benefits it is touted for – e.g., engendering trust, increasing understanding, and promoting algorithmic safety and reliability.
Speaker
For information, please contact:
Email: datascience@hku.hk